The Ultimate Guide to Data Annotation: Unleashing Its Business Potential
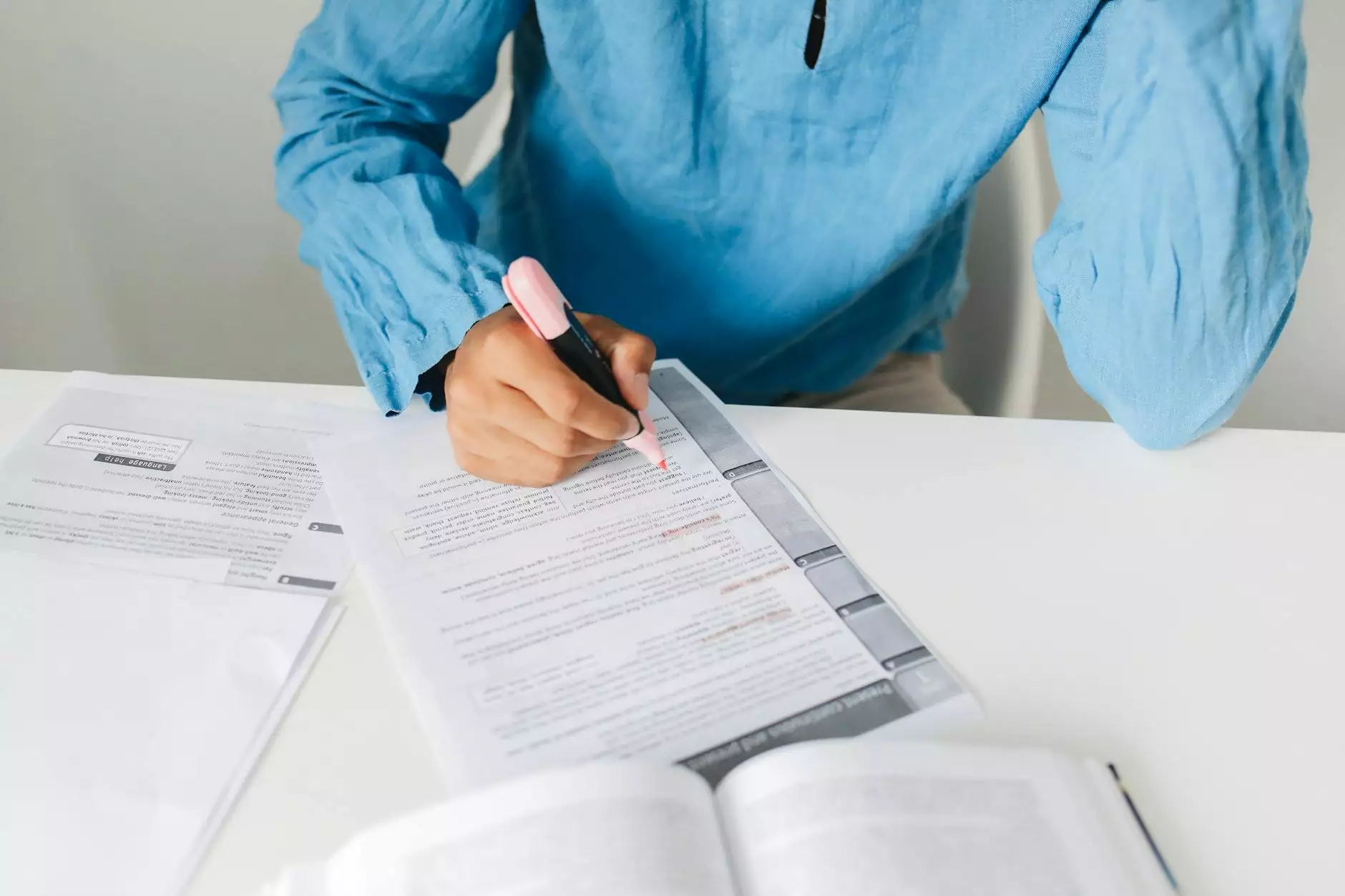
In the rapidly evolving digital landscape, data is often described as the new oil. However, this oil requires significant refinement to become valuable. This is where data annotation plays an essential role. In this article, we will explore the various facets of data annotation, its importance in business, and how it supports the development of advanced technologies such as artificial intelligence (AI) and machine learning (ML).
Understanding Data Annotation
Data annotation is the process of labeling data to make it understandable by machine learning algorithms. This labeling enables computers to learn from the data and make predictions or decisions based on that information.
Data can come in various forms, including images, text, audio, and video. Annotating this data means adding meaningful metadata that describes or classifies the data, making it a vital resource for training AI models.
The Importance of Data Annotation in Business
Incorporating data annotation within your business strategy can lead to significant advancements in productivity and innovation. Here are several reasons why it is crucial:
- Enhanced AI Performance: Well-annotated data leads to higher accuracy in machine learning models. The more precise the annotation, the better the outcomes.
- Cost Efficiency: By utilizing annotation tools, businesses can save time and resources. Automated solutions allow even manual annotations to be done quickly and accurately.
- Scalability: Businesses can scale their operations more effectively with a ready supply of annotated data, essential for growing AI applications and services.
Types of Data Annotation Methods
Various methods can be employed for data annotation, depending on the data type and the intended application. These include:
1. Image Annotation
Image annotation involves tagging images with labels that indicate the content within the image. This includes bounding boxes, segmentation, and landmarking. It is extensively used in computer vision tasks for applications such as facial recognition and object detection.
2. Text Annotation
Text annotation entails the process of labeling text with relevant tags. This may involve entity recognition, sentiment analysis, or part-of-speech tagging. This type of annotation is essential for natural language processing applications that require the understanding of human language.
3. Audio Annotation
Audio annotation is the process of transcribing and tagging audio clips to provide context. This is important for applications like voice recognition, where clarity and accuracy are paramount.
4. Video Annotation
Video annotation involves annotating frames within videos for various analysis types including activity recognition and event detection. It is crucial for developing applications that require real-time action identification.
Choosing the Right Data Annotation Tool
Selecting the right data annotation tool can greatly influence the quality and efficiency of the annotation process. Below are key factors to consider:
- Type of Data: Ensure the tool supports the specific data type you will be annotating, whether it's text, images, or audio.
- User-Friendliness: The tool should have an intuitive interface that simplifies the annotation process, making it easy for team members to use.
- Collaboration Features: Look for tools that allow multiple users to work simultaneously and track changes effectively.
- Integration Capabilities: The tool should integrate seamlessly with existing systems and workflows within your organization.
- Quality Control: Evaluate the tool's features for maintaining data quality, including review and validation processes.
Top Data Annotation Platforms
When it comes to data annotation, various platforms provide comprehensive solutions. Here are some noteworthy options:
1. Keylabs.ai
Keylabs.ai offers a powerful data annotation platform that streamlines the process of labeling diverse datasets. Their tools are equipped with AI-driven features that enhance consistency and accuracy, making it an ideal choice for businesses seeking high-quality annotations at scale.
2. Amazon SageMaker Ground Truth
A cloud-based service that provides a robust framework for building, training, and deploying machine learning models. It minimizes the cost of labeling by integrating human and machine learning workflows.
3. Labelbox
Labelbox is a collaborative data annotation platform designed for digital and AI teams. Its customizable workflows make it adaptable to various annotation projects and types.
4. Supervisely
This platform boasts a suite of tools for the computer vision community, offering user-friendly annotation and management services tailored to image and video data.
How Data Annotation Drives AI and Machine Learning Success
The success of AI and ML initiatives largely hinges on the quality and quantity of annotated data. Below are key ways in which data annotation contributes to success:
1. Improved Model Accuracy
Accurate data labeling leads to effective training of AI models, resulting in high-performance outputs.
2. Better Data Understanding
Annotated data offers deeper insights into data characteristics, which aids in refining algorithms and enhancing models.
3. Speed of Deployment
Efficient data annotation processes mean that businesses can roll out their AI solutions faster, staying competitive in their industries.
Challenges in Data Annotation
While the benefits of data annotation are substantial, several challenges must be addressed:
- Consistency: Variability in labeling can lead to discrepancies in training data, affecting model performance.
- Scalability: As the volume of data increases, scaling annotation efforts becomes complex and resource-intensive.
- Quality Assurance: Ensuring high-quality annotations requires robust review processes and validation techniques.
The Future of Data Annotation in Business
The future of data annotation is set to evolve with advancements in technology and methodologies. Upcoming trends to watch include:
1. AI-Assisted Annotation
Leveraging AI to automate initial annotation can significantly speed up processes while enhancing overall accuracy. These systems learn from user corrections, improving over time.
2. Crowdsourced Solutions
Utilizing a vast pool of annotated data from the crowd can enhance quality and reduce costs, especially for large-scale projects.
3. Real-Time Annotation
Recent innovations allow for real-time data annotation, which is crucial in applications such as autonomous vehicles or live video analytics.
Conclusion
As businesses continue to leverage AI and machine learning, the role of data annotation will become increasingly pivotal. With the right tools and platforms, companies can harness the power of their data, improve operational efficiency, and drive innovation. By investing in quality data annotation, organizations not only enhance their current capabilities but also set the foundation for future advancements in technology.
Explore how Keylabs.ai can be your partner in unlocking the true potential of data annotation for your business. Embrace the future of AI and transform your operations today!